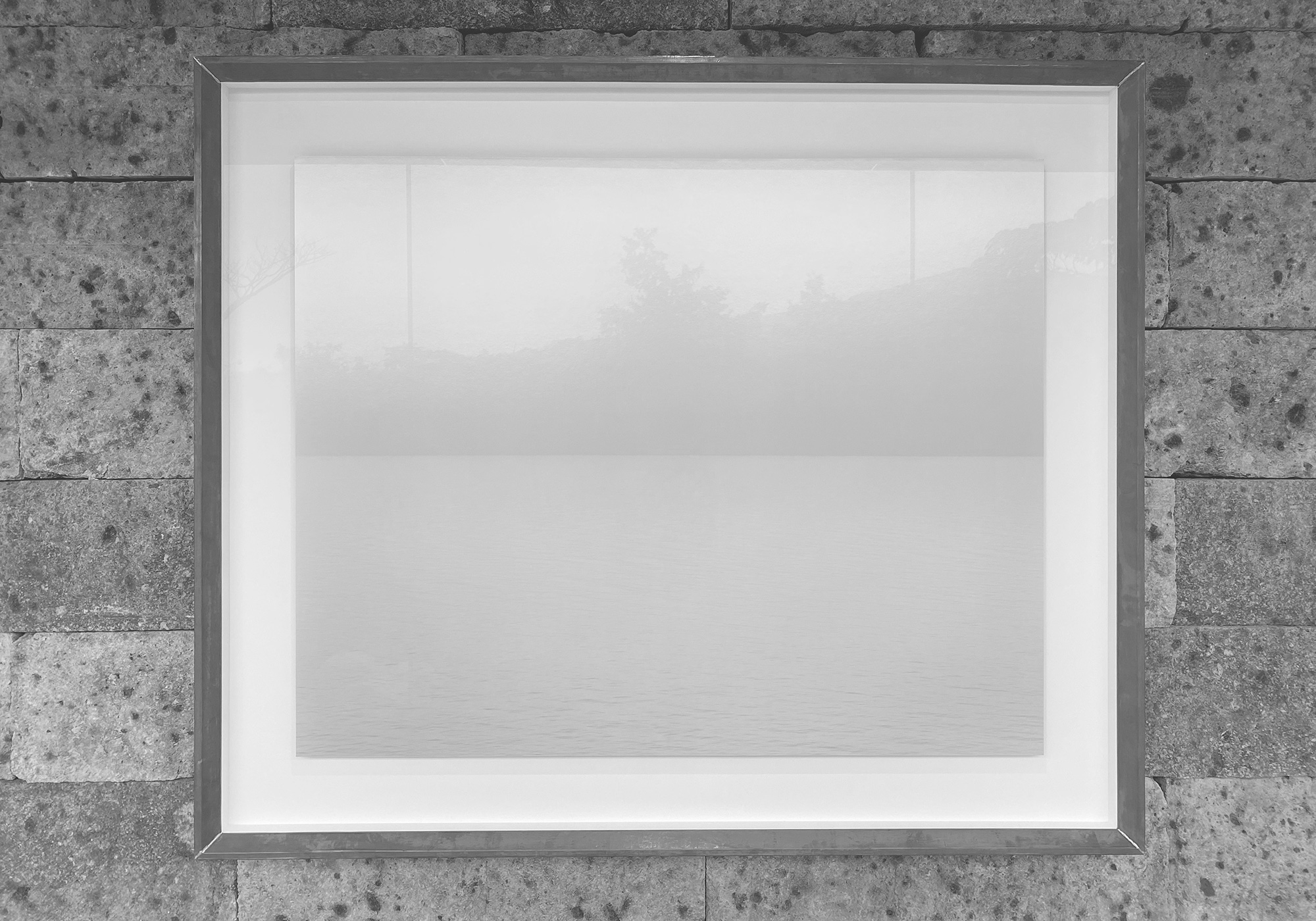
Justin Cheng studies networks and communities. He likes chocolate, and is currently a data scientist at Discord. He holds a PhD in Computer Science from Stanford University.
Justin Cheng studies networks and communities. He likes chocolate, and is currently a data scientist at Discord. He holds a PhD in Computer Science from Stanford University.